Scientific Reasoning
21 Significant Correlations and Controlled Studies
Section 1: Introduction
Science is in the business of offering explanations—more specifically, it is in the business of trying to discover the causal relations in nature. Identifying a correlation can be one step in this process, and in the last chapter we looked at correlations and how they relate to causal thinking more broadly. As we saw, although a correlation can be a good place to start, by itself a correlation never justifies drawing a causal conclusion. After all, there are many possible explanations for the existence of a correlation. Moreover, we briefly talked about some ways of ruling out alternative explanations, and thereby identifying significant correlations—or correlations that are suggestive of a causal relation. In this chapter we will look at some more sophisticated techniques for identifying significant correlations—techniques often used in science and social science. We will look at two of the most common: the observational study and the randomized controlled study. The following is not intended to prepare you to conduct your own studies—conducting a study requires expertise and resources (and time!) most of us do not have. Rather, the aim is to show the reasoning behind these studies, and to give us the tools and vocabulary to be more informed and critical readers of scientific studies.
Section 2: Observational and Experimental Studies
Suppose that you have spotted a pattern. Suppose that among your friends you have noticed that those who regularly eat breakfast have higher grade point averages (GPAs) than those who do not. This observation leads you to think that maybe you ought to eat breakfast, and maybe other students should too. Put in our terminology: among your friends, regularly eating breakfast is correlated with GPA, and this correlation may be indicative of a causal relation between the two. So, is that right—does this correlation establish that eating breakfast is causally relevant to GPA? Well no. To conclude that the eating breakfast is causally relevant to GPA is to risk a hasty explanation. Not only is this correlation based on a relatively small sample (your friends), but there are many possible explanations for any correlation, and we need to investigate the plausibility and adequacy of these alternatives before identifying a particular explanation as the best.
Nevertheless, observing this pattern gives you a place to start. In order to determine whether this pattern is suggestive of a causal relation, you would need to scale up your inquiry in a way that would allow you to (i) find a genuine correlation if there is one, and (ii) rule out, or at least cast doubt upon, some of the possible explanations for the correlation. We will discuss two techniques a researcher might use. The first technique is called an observational study. To conduct an observational study in this case, a researcher would systematically identify and examine a group of students to see whether there is a significant correlation between the two factors in question. Alternatively, a researcher might choose to conduct an experimental study. Unlike an observational study, in an experimental study researchers go beyond mere observation to intervene by systematically exposing subjects to the suspected cause. In this case, the researcher would (among other things) identify a specific group of students, give them breakfast (or not), and see what happens. How do these techniques take up or cast doubt upon alternative explanations for the correlations? Let us take a closer look one-at-a-time.
Section 3: Observational Studies
There are different kinds of observational study. A retrospective observational study begins with the effect you are interested in and looks backwards in time to try to isolate a cause. To conduct a retrospective study on the Breakfast/GPA hypothesis, you’d start with students’ GPAs and then look to see whether they eat breakfast or not. A prospective observational study, on the other hand, begins with a suspected cause and follows it forward in time to see if the effect follows. So, in the case at hand you’d start by looking at breakfast eating patterns and look for patterns in GPAs. There are other differences between prospective and retrospective studies (e.g. retrospective studies tend to be much easier to conduct), but in discussing the reasoning behind observational studies we will focus on a prospective study, though our conclusions will largely apply to retrospective studies as well.
Let us return to the case at hand. In order to dig deeper into your tentative view that breakfast contributes to GPA, you’ve decided to conduct a prospective observational study. How will this work? You’ll have to start by identifying a group of subjects. These people will constitute your sample. The larger your sample is, the less likely your results will be mere coincidence. So, you’ll want to work with the largest sample that is feasible given the limitations of time, resources, and so on. How should you choose the people in your sample? Here is a strategy that might seem intuitive: find as many students as you can who regularly eat breakfast. Once you’ve got this group, you could then simply look at their GPAs. This is too quick, however. This strategy for identifying a sample won’t work since, as we’ve seen, correlations are comparative claims. If we are looking to see whether eating breakfast is significantly correlated with GPA, we’ll need to compare the percentage of people who eat breakfast and have high GPAs to the percentage of people who do not eat breakfast who have high GPAs. That is, we need to look at two groups with respect to GPAs: the experimental group that exhibits the suspected cause and the control group that does not. Let’s call the experimental and control groups in this case the Breakfast Group and the No-Breakfast Group.
Say you have identified a reasonably large sample of students, half of which tend to eat breakfast, half of which do not. This makes it more likely you’ll find a genuine correlation if there is one, and mitigates against the possibility of coincidence. Are we ready to look at GPAs? Not yet. Remember, we want to know whether breakfast is a causal contributor to GPA. Finding a correlation between the two will not, all by itself, suggest this, since there are many possible explanations for any correlation. Recall the four types of explanation from Chapter 20. It could be that breakfast contributes to GPA as you suspect (Type 1), but it could also be the other way around (Type 2). Moreover, there could be some underlying factor that explains the correlation (Type 3), or it could be coincidence (Type 4).
The set-up of the study mitigates the possibility of coincidence, and background knowledge casts doubt on Type 2, since it seems unlikely that a GPA could influence breakfast habits. This leaves Type 3: an underlying cause. Could there be some underlying factor that accounts for a person’s breakfast habits and their GPA? Sure. Of particular importance is the possibility of one or more confounding factors (sometimes called “lurking factors”). A confounding factor for a particular study is a factor which is (i) correlated with the suspected cause under investigation and (ii) a partial cause of the effect.
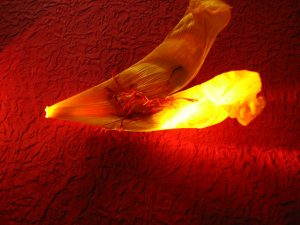
Here is an example. Suppose you were interested in the possible health benefits of the spice, saffron. To investigate this question, you might examine people who regularly eat saffron to see if they are healthier than those who never (or rarely) do. There are confounding factors here, however. Saffron is an extremely expensive spice; as a consequence, people who regularly consume saffron also tend to be relatively wealthy. Thus, there is a correlation between wealth and the suspected cause (saffron consumption). In addition, there is also a correlation between wealth and the suspected effect, since wealthy people have access to regular preventative health care at a higher rate than non-wealthy people do. Thus, if there is a correlation between saffron consumption and health, this correlation may be explained by people’s wealth—not because saffron has any health benefits. That is, wealth is a confounding factor in the effort to determine whether saffron has health benefits.
Let’s return to the question of whether eating breakfast is causally relevant to GPA. How could we conduct our study to avoid confounding factors? In brief, we will need to (i) think about the kind of factors that may be causally relevant to GPA, and (ii) make sure we take this into account in deciding how to construct the Breakfast and No-Breakfast groups. Given this, let us consider factors that might influence a person’s GPA. One prominent determinant of a student’s GPA, for example, is the difficulty of their course schedule. In some classes it is more difficult to get an ‘A’ than in others. To simplify, let us pretend that there is only one class like this—say Organic Chemistry. In addition, imagine that 10% of the students in the Breakfast Group, but none of the students in the No-Breakfast Group, are enrolled in Organic Chemistry. In this case, we would have a confounding factor, since being enrolled in Organic Chemistry is causally relevant to a student’s GPA and is correlated with the suspected cause (eating breakfast).
Why does this matter? Well, if we don’t somehow account for students’ course schedules, our results might not tell us anything definitive about whether eating breakfast is relevant to GPA—even if it is! Here is why: say that we find no difference in GPA between the groups. The natural inference here would be that eating breakfast—at least within this group of students—had no effect on GPA. But if the groups differ in this way, it may be that that eating breakfast really did have an effect, but that this effect has been hidden or masked by the unusually low grades coming from the students enrolled in Organic Chemistry. Put in different terms, if eating breakfast is relevant, then you wouldn’t know it, since its effects on GPA are mixed together or confounded with the effects of course difficulty. In order to determine if eating breakfast contributes to GPA we need to isolate it from other causes, so that we can spot its effects (if there are any).
In order to prevent this kind of confounding, we need to take account of, or control for, the difficulty of students’ courses. In general terms, to control for a particular factor or variable is to ensure that there is no difference with respect to that variable between the two groups you are comparing. This allows you to isolate the potential effects of the factor you are interested in. To control for the difficulty of students’ courses in this case would mean making sure that the Breakfast and No-Breakfast groups are as similar as possible with respect to the number of students enrolled in Organic Chemistry. Similarly, to control for wealth in the saffron study discussed above would mean looking at two groups of equally wealthy people—some of whom regularly eat saffron, some of whom do not.
Section 4: Limitations of Observational Studies
Observational studies, even when they control for known confounding factors and study large samples, do not always give accurate results. Perhaps the chief problem is that observational studies like this cannot account for the effects of unknown confounding factors. In other words, an observational study cannot rule out all the competing explanations for the phenomena in question.
Take the case discussed above; suppose we have conducted our study in a way that controls for a variety of relevant factors (including course difficulty), and that our original suspicion has been confirmed—in this much larger and more diverse group there is a correlation between eating breakfast and GPA. While this gives us stronger reasons to think that this correlation is not mere coincidence, and further that eating breakfast is causally relevant—we cannot be sure this is the case. The problem is that there may be unknown confounders out there. For example, it may have not occurred to you that getting up early might be causally relevant to a person’s GPA. Let us assume for a moment that this is right, and that, further, people who get up early tend to eat breakfast. If we haven’t controlled for getting up early, then the results of our observational study will suggest that eating breakfast is causally relevant when, in reality, it is not.
A well-known case illustrating the limitations of observational studies involves hormone replacement therapy. In the 1990s a number of professional observational studies correlated hormone replacement therapy with a decreased risk of heart disease in post-menopausal women. Scientists had a good sense of how this replacement therapy might work, and as a result many doctors recommended this to their postmenopausal patients. However, in the early 2000s it became clear that there must be a confounding factor at work in these studies. A large randomized controlled experiment showed that that though hormone replacement therapy could have positive health benefits for some women, it did not prevent heart disease and was actually associated with a number of negative health outcomes. As it turned out, overall health was the confounding factor. On balance, healthy women were more likely to pursue and be prescribed hormone replacement therapy than their unhealthy counterparts. Since a person’s overall health is causally relevant to the chance of developing heart disease, this was a confounding factor.
This shows that observational studies have their limits, and that there are alternative methods for ruling out competing explanations. Let us take a closer look at the kind of study which ultimately revealed the flaw in this observational study—the randomized controlled experiment.
Section 5: Randomized Controlled Experiments
In well-conducted observational studies researchers make careful choices about what populations to observe. While this is also true in an experimental study, a researcher conducting an experimental study goes one step further by manipulating the suspected causal variable. Consider the breakfast/GPA case discussed above. In an observational version of this study, you divide students into two carefully chosen groups—the Breakfast and No Breakfast groups—and then check to see whether students who eat breakfast end up having better GPAs than those who do not. In contrast, in an experimental study the researcher chooses which students will eat breakfast (the experimental group) and which will not (control). There are different kinds of experimental study, but here we will focus on a particularly important one: the randomized controlled experiment or trial. As one author recently put it, “The randomized controlled trial…is one of the simplest, most powerful, and revolutionary tools of research.”[1] In this section we will explain what a randomized trial is, and why it is so powerful.
By their very design experimental studies can cast doubt on many of the possible explanations for a correlation. First, like an observational study, an experimental study takes a systematic look at a wide body of information, and in doing so limits the possibility of sheer coincidence (Type 4 explanations). Second, given a correlation between Xs and Ys, an experimental study can rule out the possibility that Ys are causing the Xs instead of vice versa (Type 2), since causes precede effects. In an experimental study the researcher introduces the suspected causal factor (X) to subjects in which the suspected effect (Y) is absent. So if the effect is subsequently observed in the population you can be sure that this is not a case of Ys causing Xs.
As we have seen, observational studies are always subject to the possibility of unknown confounders. However, the possibility of confounders, known and unknown, is greatly limited by a process of randomization—hence the value of the randomized controlled experiment. But wait. What, exactly, is randomized, and how does this mitigate worries about confounders?
What makes an experiment like this randomized is that the individuals or members of the experimental group and the control group are chosen randomly from a targeted population. To do so, a researcher uses a procedure that gives each member of the population an equal chance of being chosen. So, in this case you might assign students a number and use a random number generator that is available online to assign individuals to each group. Ok, but how does randomizing who is chosen for each group mitigate against confounders?
Let us return to the unknown confounder considered in the breakfast case: for the sake of argument let us assume that it is not eating breakfast, but getting up early that is causally relevant to GPA (perhaps because they are more alert during morning classes). Because there are more people who get up early in the Breakfast Group than in the No-Breakfast Group your observational study will mistakenly suggest that breakfast is causally relevant to GPA. However, in a randomized controlled experiment this is much less likely. How so?
By randomly assigning students to either the experimental group or the control group, you would likely distribute early risers evenly into both groups (at least roughly). Randomizing in this way breaks the problematic relation between breakfast eaters and early risers, and allows us to see more clearly whether eating breakfast, in itself, has any effect. The same will go for other unknown confounders; after all, arbitrarily splitting subjects into the control and experimental groups will likely distribute other possible confounders evenly (at least roughly) between the groups. In other words, by randomly assigning subjects to the experimental and control groups the researcher will thereby automatically control for unknown confounders.
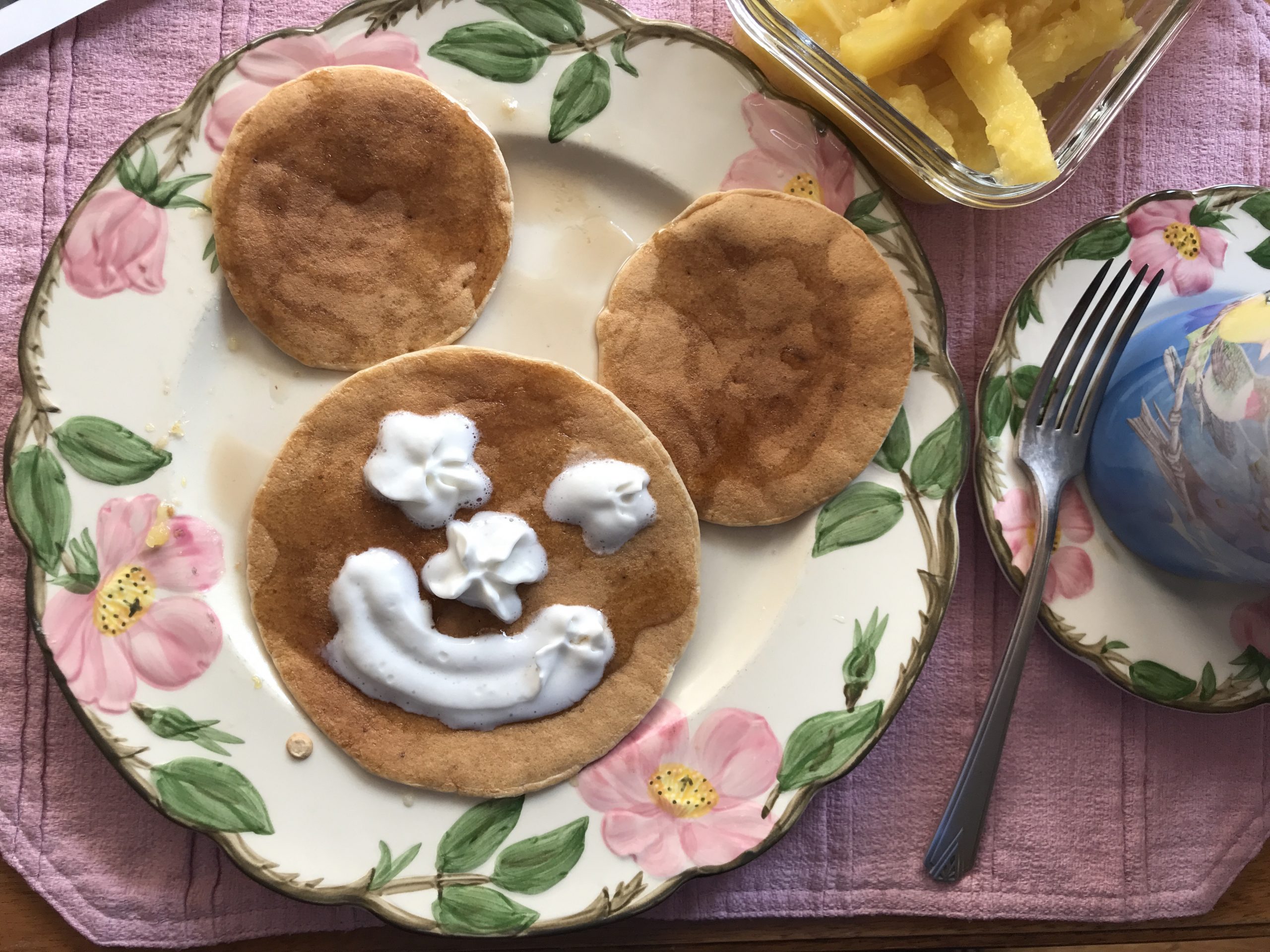
This is the chief benefit of a randomized controlled experiment—it reduces the chances that an unknown confounder will bias your results. Unfortunately, however, a study of this kind does not completely eliminate this possibility. It is always possible—even with a random assignment of subjects—that some causally relevant factor will end up accidentally associated with the experimental group. Even so, a randomized controlled experiment is much more likely to give you an accurate picture of the relationship between two (or more variables), and these studies are considered the single best way to determine causal relationships.
Section 6: Limitations on Experimental Studies
The comparison above between different kinds of experimental techniques raises a question: if randomized controlled experiments are the best way to identify significant correlations and causal relations, why do researchers ever do any other kind of study? First off, randomized controlled experiments are time consuming and can be quite expensive to conduct. Second, in some cases it is not practical or ethical to do a randomized controlled experiment. To illustrate, consider the disease known as Ebola. Ebola is a viral hemorrhagic fever that carries a high risk of death for which there is no known cure. However, there are a number of experimental treatments. The problem is that in order to do a randomized controlled study for one of these treatments, researchers would need to set up a control group that received only a placebo. In this case, the control group would not get the possible benefits of the treatment, which in this case might realistically include survival.
This point generalizes, in that when a person’s life is at stake, an experimental study can be simply unethical. This point was made in a (somewhat) humorous way in an article from the British Medical Journal:
“As with many interventions intended to prevent ill health, the effectiveness of parachutes has not been subjected to rigorous evaluation by using randomized controlled trials. Advocates of evidence based medicine have criticised the adoption of interventions evaluated by using only observational data. We think that everyone might benefit if the most radical protagonists of evidence based medicine organized and participated in a double-blind randomized placebo controlled, crossover trial of the parachute.”[2]
Conducting a randomized study of the effectiveness of parachutes using real people would be obviously unethical, and so too when it comes to other potentially life-saving or life interventions.
A third consideration is that simply because randomized controlled studies are the single best way to uncover significant correlations, does not mean that observational studies have no value. Observational studies tend to be more economical in terms of time, effort, and resources than experimental studies, and so are useful (among other things) for studying a hypothesis in a preliminary way—as a way of deciding whether it is worth using the resources to conduct a randomized controlled experiment. Moreover, when multiple observational build upon one another and largely concur in their conclusions, then they can give us good reason to endorse causal conclusions.
Exercises
Exercise Set 21A:
Directions: Consider the following possible studies. What confounding factors might you need to control for?
#1:
A study to assess whether being an early reader (a person who learns to read before age 5) causally contributes to academic success.
#2:
A study to assess whether fluency in a second language improves scores on standardized tests.
#3:
A study to assess whether taking multi-vitamins prevents chronic illness.
#4:
A study to assess whether listening to classical music while studying makes it easier to remember information.
Exercise Set 21B:
#1:
Comment on the following experiment:
A vitamin company has developed a new pill intended to prevent strep throat (a kind of bacterial infection). The company claims that they pill was given to 2000 people daily for a month, and only 3% of subject came down with strep throat during this time. On the basis of this experiment, the company sells the vitamin as a preventative.
#2: What kind of study is the following, and what do you make of the study itself?
A researcher is interested in investigating whether pet ownership contributes to lower blood pressure. The researcher identifies 30 people who have pets and 30 people who do not. She takes everybody’s blood pressure several times, and then averages the results. It turns out that the pet owners in the researcher’s sample have lower blood pressure than the non-pet owners. The researcher takes this to be good preliminary evidence of a causal connection.
#3:
In his book Exercised: Why Something We Never Evolved to Do is Healthy and Rewarding, Daniel Lieberman comments on a study of physical activity and health. He summarizes and evaluates the study as follows.
“Researchers put accelerometers “on a diverse sample of eight thousand Americans above the age of forty-five and then tallied up who died over the next four years—about 5 percent of the sample. Predictably, those who were more sedentary died at faster rates, but these rates were lower in people who rarely sat for long uninterrupted bouts….One flaw with this study is that people who are already sick are inherently less able to get up and be active.”[3]
What kind of study is Lieberman commenting on, and what correlation did it find? Also, explain Lieberman’s criticism of the study.
#4:
What kind of study is the following, and what do you make of the study itself?
A researcher wants to study whether taking notes by hand is more effective than typing notes when it comes to remembering them. He identifies 30 students who are willing to participate, and allows them choose whether they’ll be in the experimental group or the control group, and the groups end up even (15 people in each). The researcher then requires students to attend the same hour-long lecture. One group takes notes by hand, the other takes notes by typing on their laptops. Students are allowed to study their notes and are given a test over the lecture 3 days later. When the researcher crunches the numbers, it turns out that students in this population who took notes by hand got higher grades than those who took notes on a laptop.
#5:
Come up with one experiment you’d love to know the results of—if you had the time, money, and expertise to do so. What would it be, how you set it up, and why?
- Jadad, Alejandro R. and Enkin, Murray W. (2007). Randomized Controlled Trials: Questions, Answers, and Musings 2nd ed. Malden, MA: Blackwell, 1. ↵
- Smith G. C. , Pell, J. P. (2003). "Parachute use to prevent death and major trauma related to gravitational challenge: systematic review of randomised controlled trials." BMJ. 327(7429):1459-61. ↵
- Lieberman, Daniel E. (2020). Exercised: Why Something We Never Evolved to Do is Healthy and Rewarding. New York: Pantheon Books, 66-67. ↵